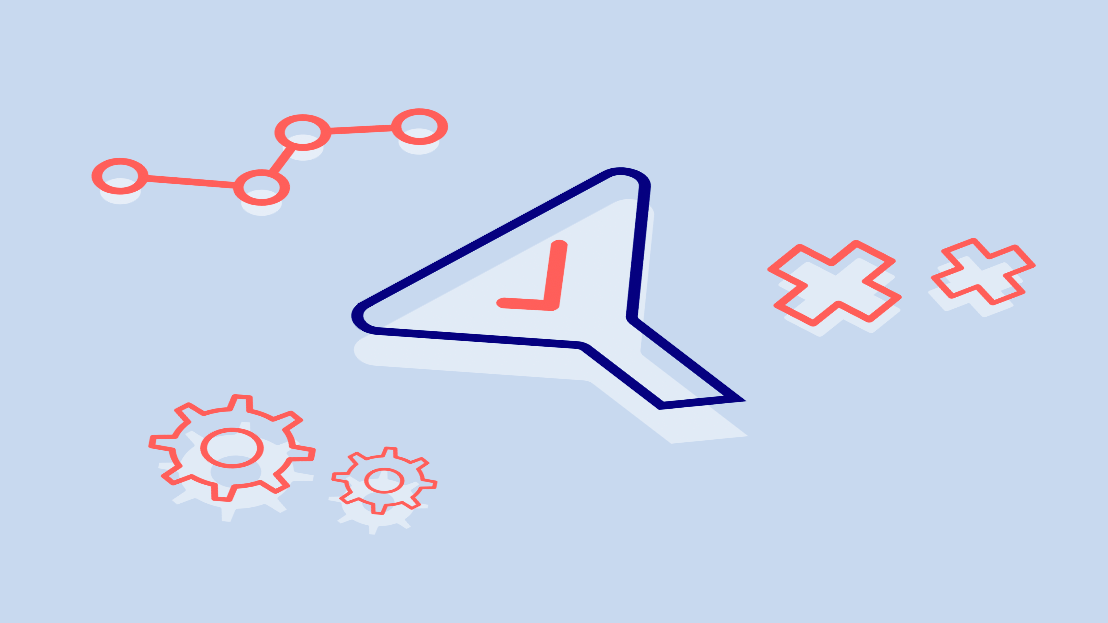
Leveraging the OHDSI OMOP common data model for research using observational health data
Leveraging the OHDSI OMOP common data model for research using observational health data
When it comes to research, a clinical common data model is an important part of any health organisation. Collaborative research and data sharing, with appropriate security and data privacy measurements on the common data model, empowers organizations to work together to improve patient outcomes.
Data4Life’s Analytics Platform for Research leverages the Observational Health Data Sciences and Informatics (OHDSI) Observational Medical Outcomes Partnership (OMOP) common data model (CDM) to enable standardized analysis across different data modalities on collaborative research and data sharing.
What is OHDSI?
The OHDSI program is a multi-stakeholder, interdisciplinary organization. It creates open-source solutions that bring out the value of observational health data through large-scale analytics.
The OMOP CDM and vocabulary aim to bring together disparate observational health databases to support common analysis.
The benefits of working with OHDSI and the OMOP CDM are the following:
- Once data is mapped to the OMOP CDM, you can conduct faster and more reliable studies across a series of datasets and data types.
- OHDSI provides tools for data analysis, reducing costs for developing core software components to access the data.
- The OHDSI network supports result exchange in remote multi-center database studies without having to share the actual data.
How it works
Various clinical data modalities are transformed to fit into a CDM that allows queries, analysis, and studies to be developed once and deployed in the OMOP CDM database.
The OMOP CDM provides flexibility thanks to an optimized schema. There are tables for data typically required in clinical trials and observational studies, such as drug use and procedures performed. These tables are grouped in the clinical data part of the clinical data warehouse. For some use cases, extending the current OMOP CDM might be required.
Furthermore, the OMOP CDM includes the major commonly used ontologies such as SNOMED CT, RxNorm, LOINC, and so on.
All details of the OMOP CDM are described on the OHDSI wiki pages.
You'll need to understand your source data well before performing the extract, transform, and load (ETL) to the OMOP CDM. Once the data is in the OMOP CDM database, the data is ready to be used for your study.
The following overview shows this process end-to-end. Clinical data is extracted, transformed, and loaded to CDM and extended data model with several outcomes, such as advanced analytics and data science.
The software tools that OHDSI provides are described on this OHDSI software tools page.
The Data4Life Analytics Platform for Research offers the following OMOP tools and services:
- Observational health data modelled in a standardized format
- Extension of OMOP CDM to fit into specific use cases
- Software and analytics tools for OMOP CDM
Data4Life – helping researchers and scientists analyze data
OHDSI and the OMOP CDM open-source solutions bring out the value of observational health data through large-scale analytics.
The Data4Life Analytics Platform for Research leverages these solutions to offer researchers and scientists the tools to generate high-quality and reproducible evidence from observational health data. The data can be extracted, transformed, and loaded from various source systems such as the electronic health record, patient management, and others.